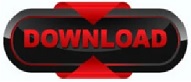

Of course, that increases the complexity of the annotation task. In the context of question answering, labels are swapped for answer spans.

Clear and comprehensive annotation guidelines facilitate the annotator’s job. When annotating, you’ll struggle through cases where multiple labels might fit an example - or none at all. Real-world complexities often prevent neat categorization into discrete labels. But anyone who has annotated would say otherwise. Annotating Natural LanguageĪnnotation sounds like an easy enough task: simply assign a label to a sentence, paragraph, or document.
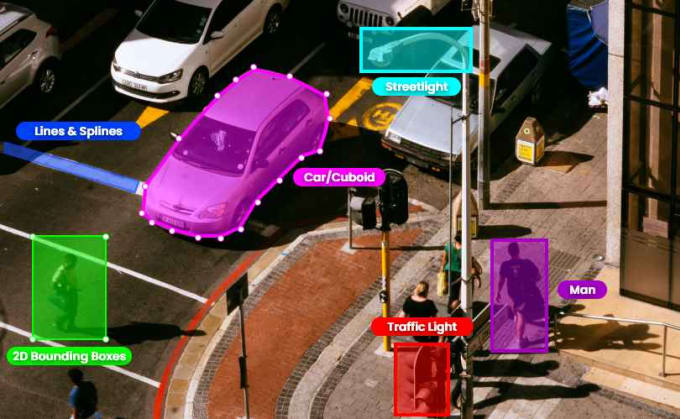
It involves identification of raw data, e.g., images, videos, text documents, and assigning labels to that data so that the machine learning model can properly interpret the context, learn from it and make accurate prediction during the inference process.įor example, labels might ‘tell’ whether an image contains a dog or train, which words an audio recording consists of, or if a text file contains answers to a specific question.ĭata labeling is needed for many use cases where machine learning is involved, such as computer vision, speech recognition, or natural language processing. What is Data Labeling?ĭata labeling (or ‘annotation) is one of the tasks necessary when developing and maintaining a machine learning model.
#DATA LABELLING AND ANNOTATION HOW TO#
Read on to learn more about Haystack annotation tool and how to use it.
#DATA LABELLING AND ANNOTATION FREE#
Haystack provides a free annotation tool to assist you in creating your own question answering (QA) datasets, making the process quicker and easier. But creating high-quality datasets from scratch is a tedious and expensive process. Supervised models depend on labeled data for both training and evaluation.
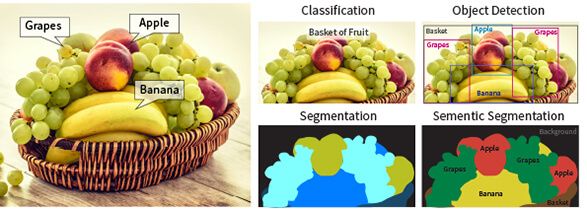
This is especially true for Transformer-based neural networks, which are particularly apt for solving natural language tasks - be it question answering (QA), sentiment analysis, automated summarization, machine translation, or text classification. Without annotated datasets, there would be no supervised ML. Over time, the model can label more and more data automatically and substantially speed up the creation of training datasets.If you’re interested in natural language processing (NLP), then you are probably aware of the importance of high-quality labeled datasets to any machine learning model. The human-generated labels are then provided back to the labeling model for it to learn from and improve its ability to automatically label the next set of raw data. Where the labeling model has lower confidence in its results, it will pass the data to humans to do the labeling. Where the labeling model has high confidence in its results based on what it has learned so far, it will automatically apply labels to the raw data. In this process, a machine learning model for labeling data is first trained on a subset of your raw data that has been labeled by humans. To overcome this challenge, labeling can be made more efficient by using a machine learning model to label data automatically. The majority of models created today require a human to manually label data in a way that allows the model to learn how to make correct decisions. But, the process to create the training data necessary to build these models is often expensive, complicated, and time-consuming. Successful machine learning models are built on the shoulders of large volumes of high-quality training data. In machine learning, a properly labeled dataset that you use as the objective standard to train and assess a given model is often called “ground truth.” The accuracy of your trained model will depend on the accuracy of your ground truth, so spending the time and resources to ensure highly accurate data labeling is essential. The machine learning model uses human-provided labels to learn the underlying patterns in a process called "model training." The result is a trained model that can be used to make predictions on new data. The tagging can be as rough as a simple yes/no or as granular as identifying the specific pixels in the image associated with the bird. For example, labelers may be asked to tag all the images in a dataset where “does the photo contain a bird” is true. Data labeling typically starts by asking humans to make judgments about a given piece of unlabeled data. For supervised learning to work, you need a labeled set of data that the model can learn from to make correct decisions. Today, most practical machine learning models utilize supervised learning, which applies an algorithm to map one input to one output.
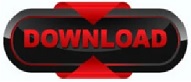